June 2023 Community News!
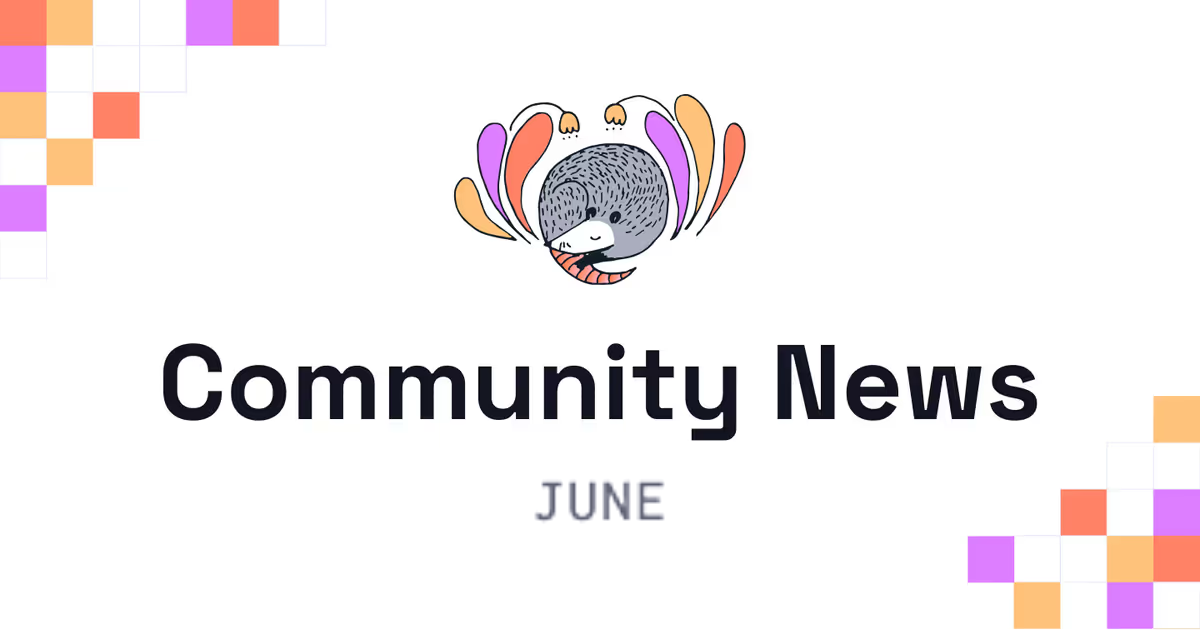
đź”¶Â Heartex is now HumanSignal
The HumanSignal team behind the Label Studio open source project and Enterprise platform takes a leap forward with a new name that matches our core belief that the future of AI is not just about complex algorithms or massive computing power: It is about people. It is about the signal that humans provide, which powers these models, helping them to adapt, learn, and align with the needs of organizations and society at large.
Label Studio, which has enabled more than 250,000 data scientists and annotators to label 200+ million pieces of data, will remain at the core of our mission. We'll focus on building features and products to continually increase the quality and efficiency of human feedback—or signal—helping you take advantage of new foundation models and methodologies rapidly emerging in this space.
🚀 Label Studio 1.8 Release for Fine-Tuning LLMs
Our latest release, Label Studio 1.8, is designed with fine-tuning Large Language Models (LLMs) in mind. Create datasets to easily retrain LLMs like ChatGPT or LLaMA with new templates and workflows, including our new Ranker interface to easily organize your annotation workflow through ranking and categorizing annotation predictions.
Learn how you can get started with the new Ranker Interface, other new Generative AI labeling templates, and many other user experience improvements.
{🤖} Understanding the Label Studio JSON Format
Ever wonder how our JSON format is generated, or how to structure your JSON files to import data, pre-annotate your datasets, or work with the ML backend? Wait no further! Our latest post on the blog breaks down our JSON format and what to look for when working with it.
Case Study: Outreach
Curious how to improve your data process and efficiency all in one go? 🤔
Read how Outreach reduced development time for new labeling tasks by 25% with the help of Label Studio.
Community Shoutouts 🎉
Thank you to Brad Neuberg and the Planet Labs team for inviting us to their office and to Rizèl Scarlett for hosting us on GitHub’s Open Source Friday!
Shoutout to those who tuned into our events this month and gave us helpful feedback, including Jeremy Moore, Duco Gaillard, Claire Longo, Youngwan Lim, and LoĂŻc Dugast.
Annotations
We’re loving the recent analysis from Casey Newton’s Platformer Newsletter on the impact that LLMs and other foundational models could have on the future of data annotation and model building.
- Crowd-sourced annotation emerged in the early 2000s as a reliable way to collect high-quality, human-generated data, and was partially responsible for helping usher in the new wave of powerful deep-learning models — but what does the future hold for human-sourced annotation with the rise of LLMs? Researchers at EPFL reported that 33-44% of crowd-sourced annotators were using LLMs to complete their tasks. LLMs are now forcing many data-annotation teams to rethink how they crowd-source tasks.
- (On a related note, this change in crowd-source annotation is reflected anecdotally in an article on the annotation economy in New York Magazine. It’s a fascinating look at the world of data labeling that ended with a description of how one crowd-sourced annotator “decided to stop playing by the rules… and gets high marks for quality… thanks to ChatGPT.”)
- This shift in data collection and annotation has important implications for future model development. A group of researchers from the University of Oxford, University of Cambridge, University of Toronto, and Imperial College London discovered "the use of model-generated content in training causes irreversible defects in the resulting models.” As ML-generated content becomes more widely used and available, knowing the provenance of training data will become increasingly important to protect model integrity. Caution must be exercised when using ML models to ensure the integrity of the original data.
And finally…
- It’s no secret that we’re big fans of Reinforcement Learning from Human Feedback (and in case you missed it, check out the talk the Label Studio team delivered at PyData Berlin). If you’ve wanted a deeper dive into the subject, Nathan Lambert has published a new explainer on How RLHF Actually Works. It’s worth a read if you want to know more about this essential technique for tuning LLMs.