Tales from Our Community: Stop the Traffik
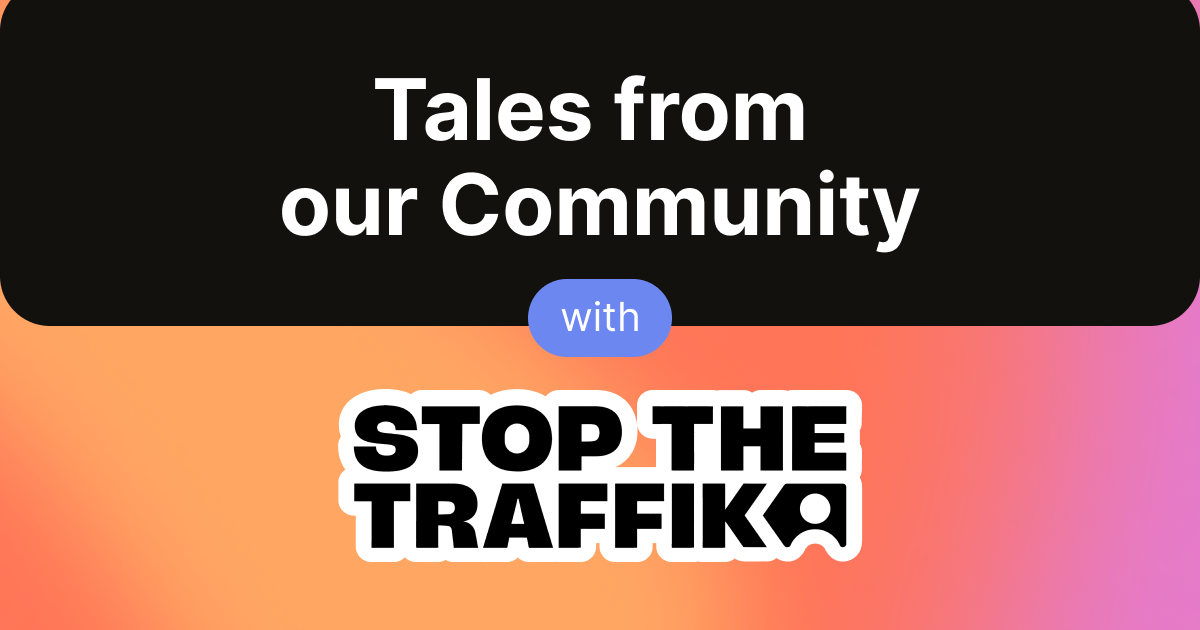
The Challenge: Turning Raw Data into Actionable Intelligence
Human trafficking is one of the hardest crimes to track. Traffickers work in the shadows, victims often can’t speak out, and the people who could intervene only see scattered pieces of the bigger picture.
Stop the Traffik gathers and analyzes data from different sources like news reports, blogs, articles, social media, and frontline organizations to uncover trafficking patterns. Their platform, the Traffik Analysis Hub, processes and produces key insights from hundreds of thousands of reports, using Machine Learning and Generative AI. But for artificial intelligence to make a real difference, the models behind it need high-quality training and testing data.
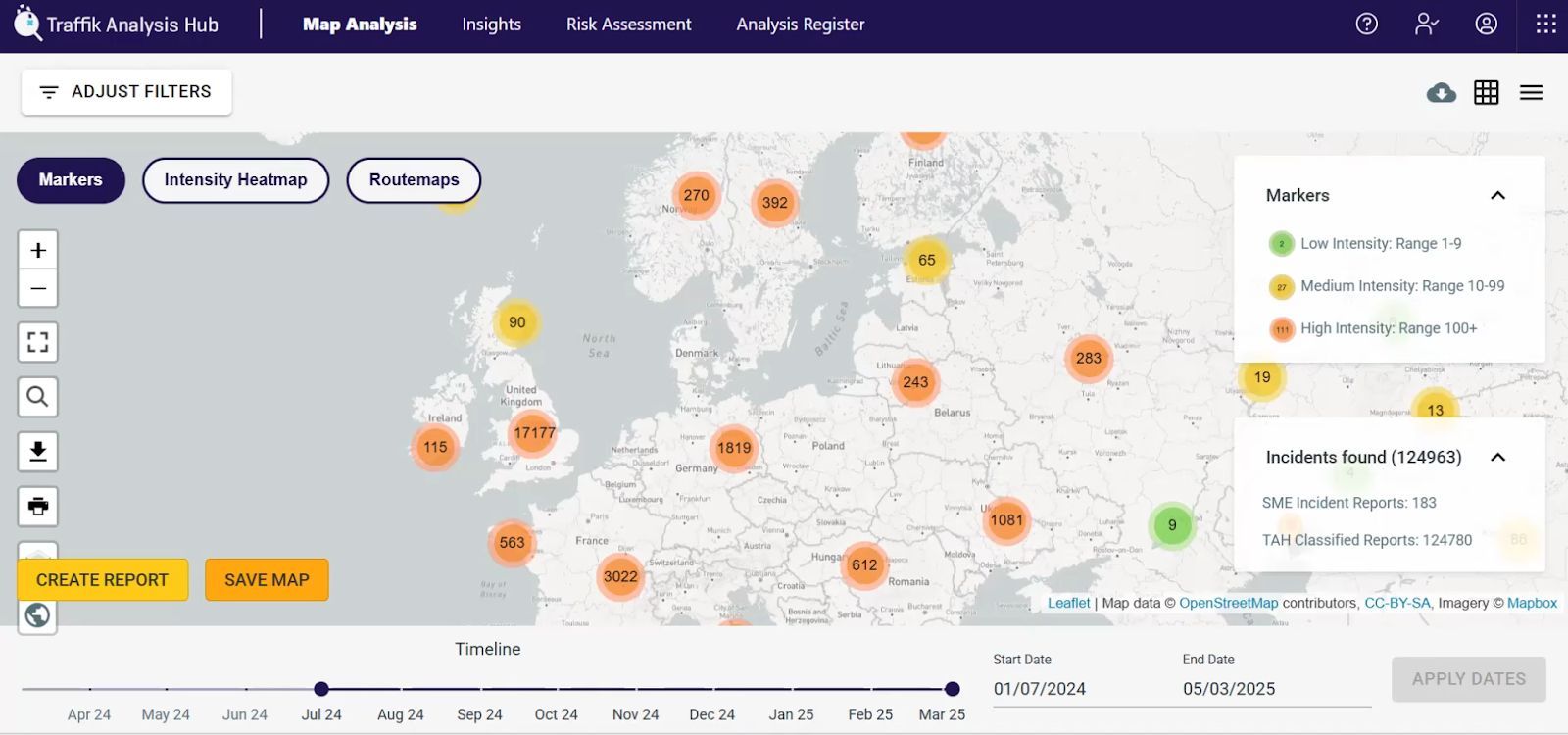
The original training data used to build their models was lost, which meant the team had to start from scratch. They had years of articles, but none of them were labeled. The first solution was a manual process—volunteers answered questions in online forms, tagging each article with key details like locations, transportation methods, and sources.
At first, this approach seemed workable. But as the team scaled up, problems became obvious. Volunteers interpreted questions differently, leading to inconsistent labeling. Some missed key details, while others added unnecessary information, making the data messy and unreliable. Because everything was done through forms, reviewing and fixing mistakes became a slow, manual process that wasn’t sustainable.
Some of the biggest challenges came from extracting implied information, for example, identifying the gender of a trafficker or victim. Reports rarely state this directly. Instead, an article might say, “Steve did this, and he was seen…” Volunteers had to recognize that "he" implied Steve was male and manually tag that information. Doing this across thousands of reports took time and was easy to overlook. Additionally, it would be practically impossible to manually identify the exact indexes of the entities required for NER tasks.
The team needed a better way to label data, one that was accurate, flexible, and easy for volunteers to use.
Why Other Tools Fell Short
Kyle, a developer from Diegesis, collaborating with Stop the Traffik, researched more than a dozen annotation tools. Prioritizing tools with advanced annotation capabilities, they focused on those that could seamlessly integrate external tools and plugins—especially LLMs—while remaining user-friendly. It was essential that these tools allowed for properly structured data, enabled easy task assignment, facilitated efficient data collection, and were either low-cost or free whenever possible.
Another problem was flexibility. A lot of tools were built for simple tasks, like labeling images with a single tag. That wasn’t what Stop the Traffik needed. Their work involved extracting multiple types of information from long reports, assigning multiple labels per article, capturing meta deta, integrating guidelines and capturing complex relationships between entities. Very few platforms could handle that level of customization.
Managing volunteers added another layer of complexity. People joined and left regularly, so they needed a tool that made onboarding easy while keeping data secure. Most platforms didn’t offer the right level of access control, forcing the team to manage permissions manually.
Kyle summed up the problem: "We needed something flexible, well-documented, and easy to use. Every tool we looked at was either too rigid, too expensive, or lacked the community support we needed."
Why Label Studio Stood Out
After testing multiple options, Stop the Traffik chose Label Studio because it met every requirement.
Unlike other platforms that forced users into rigid workflows, Label Studio gave them full control over their labeling process. They could define custom categories, format question structures, manage role based access for different people, adjust annotation workflows, and structure their data exactly how they needed.
Artificial intelligence-assisted pre-labeling speed up the process. Instead of tagging everything manually, artificial intelligence scanned reports, highlighted key terms, and suggested labels. Volunteers then reviewed and refined the results, saving hours of work.
The ability to extract implied information made a huge difference. Instead of manually identifying pronouns like “he” or “she” and linking them to specific individuals, volunteers could highlight and tag references directly in Label Studio. The system stored this metadata, making it easier to analyze patterns later.
Documentation and community support also set Label Studio apart. Unlike other tools that lacked an active user base, Label Studio had a strong, responsive community. Whenever the team ran into challenges, they found answers quickly, either through documentation or community discussions.
Kyle explained the impact: "Setting up Label Studio was incredibly intuitive. The community and documentation made a huge difference. Other tools either lacked key features or were way too expensive. Label Studio was the perfect fit."
The Impact: Faster, Smarter Data Labeling
Before using Label Studio, labeling reports meant reading through each one, copying information into spreadsheets, and fixing mistakes along the way. Now, artificial intelligence does an initial pass, identifying key details and pre-labeling articles. Volunteers then review and refine those labels instead of starting from scratch.
Accuracy has improved because artificial intelligence highlights critical information like names, locations, and types of exploitation. Volunteers work within a structured system rather than an open-ended spreadsheet, reducing errors and making their contributions more meaningful.
Uploading and managing large amounts of data is also much easier. Instead of handling documents one by one, the team can import data in bulk, assign tasks, and track progress all in one place.
As Swati, a data scientist at Stop the Traffik, put it, "Before, we spent so much time structuring raw text. Now, we can focus on what really matters—getting accurate, actionable data."
Uncovering Exploitation in the Supply Chain
Fighting human trafficking requires more than tracking individual cases. It means tackling the systems that allow exploitation to persist.
As well as commercial sexual exploitation, one of the biggest vulnerabilities is the global supply chain. Forced labor is often buried deep within subcontractor networks, making it difficult for businesses to detect and prevent exploitation.
Most companies lack full visibility into their supply chains, creating blind spots where traffickers can take advantage of vulnerable workers. Stop the Traffik works with commercial partners to close this gap, integrating supply chain data with trafficking intelligence to identify hidden risks.
With Label Studio, Stop the Traffik can process large-scale supply chain data efficiently. Businesses can use risk assessment tools to analyze supplier locations, cross-reference them with known trafficking hotspots, and flag potential vulnerabilities. Instead of sifting through raw reports manually, machine learning models trained on trafficking data help surface critical patterns faster.
AI-powered annotation streamlines this process, allowing Stop the Traffik to structure data more precisely for better insights. By combining automation with human review, they ensure accuracy while scaling their efforts. This shift moves organizations from reactive responses to proactive prevention, giving them a way to address trafficking risks before they become embedded in their operations.
Through scalable AI-driven data labeling, Stop the Traffik is giving companies the intelligence they need to take action, making supply chains safer and disrupting trafficking networks before they grow.
The Bigger Picture: AI That Supports Real-World Impact
By switching to Label Studio, Stop the Traffik has made their entire data pipeline more efficient. Their machine learning models train faster, their data quality is higher, and their volunteers can contribute more effectively. Artificial intelligence reduces the manual workload while human reviewers ensure accuracy.
Stop the Traffik is also using Label Studio’s prompt engineering features to refine artificial intelligence behavior, making pre-labeling even smarter. What used to be a slow, repetitive process has turned into a seamless workflow where artificial intelligence and human expertise work together.
Kyle summed it up: "Label Studio gave us the flexibility, support, and customization we needed. It has completely changed how we process trafficking data."
With better tools, Stop the Traffik can focus on what matters most—using data to fight human trafficking and protect vulnerable people.
To help stop trafficking, check out the Traffik Analysis Hub, Traffik Analysis Hub | STOP THE TRAFFIK. If you're looking for a better way to label your own data, try Label Studio.