What will the Long-Lasting Impacts of Large Generative Models Be?
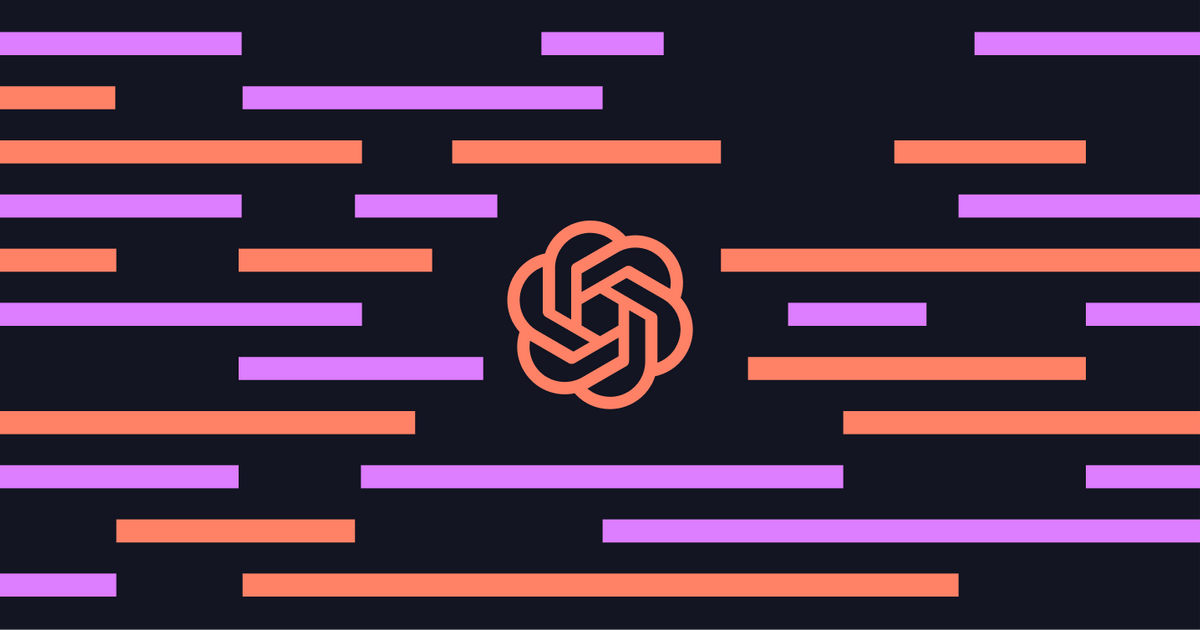
Introduction
Last week, the world excitedly got its first peek at GPT-4, OpenAI’s large foundational generative model. GPT-4 is the successor to ChatGPT, the prominent language model (LLM) that has captured the minds and imaginations of many folks in and around the tech world. It’s hard to deny the impact large-scale generative models like ChatGPT, DALL-E, MidJourney, Jasper, and Stable Diffusion have had across industries.
The generative AI market has a forecasted valuation of $53.9 billion by 2028, with impacts across retail, customer service, engineering, and research, among other industries we have yet to discover. These models – and their application – look to be the defining trend of 2020s tech. Tech behemoth Google has even seemingly scrambled to repurpose its entire development efforts to deliver on the potential that these models provide.
While many folks are heralding the progress and promise that these innovations have made — it’s hard not to be at least a little bit skeptical in the face of innovation. Some of the claims make it sound almost too good to be true.
No one has a magical looking glass or a time machine into the future (that we know of), but we do have historical knowledge of what trends in this industry have held the test of time, as well as a strong sense of what it takes to build a large-scale model.
Let's explore some of these claims by looking at this from the perspective of many open source data nerds that care about machine learning.
Evaluating GPT-4’s release through the lens of open source data science leads us to see the possibility and potential for long-term impact — but it also reiterates the underlying importance of dataset development and context provided by human feedback through processes such as reinforcement learning through human feedback (RLHF) or human-in-the-loop processes.
The importance of data in GPT-4
There’s plenty of reason to get excited about the rapid innovations in large-scale generative models. In many ways — it does feel like magic! This seamless interaction between man and machine can often feel so… human. It’s a source of surprises and delights for those working in machine learning-related fields and those using these tools for the first time.
As Brenda Laurel writes in Computers as Theatre, we can describe this type of interaction as a “mediated collaboration between designers and interactors.” We’ve created new, more accessible ways of interacting with technology, which is quite exciting. Within all of these recent developments, it’s essential to remain grounded in reality — these models are only as good as the data upon which they are trained.
If data is the fuel of these large-scale models, and the sources and process to create it is mainly unknown, then we are left with no idea of what we are filling our model’s metaphorical tank with. As Label Studio’s Head of Community, Chris Hoge, wrote in a previous article, internet-scale models often come with internet-scale biases. We all know that the internet has many dark places.
That’s not to say generative models and tools like GPT-4 aren’t essential or valuable now — these spaces are filled with excitement and wonder. Even if the only thing they accomplish is creating excitement for a rapidly-growing industry, the increase in attention and accessibility increases the number of folks working to improve the model for their best benefit.
Computer Vision for Accessibility
Part of the announcement and the live stream from OpenAI included a demonstration of the expanded capabilities of GPT-4. Specifically, GPT-4 leverages Computer Vision through Be My Eyes in a feature called “Virtual Volunteer.” At its core, the Be My Eyes tool expands on the base feature set of GPT-3 from only a text-to-text model and moves it to an image-to-text model.
Put simply: this technology can take an image that you upload and convert it into a description of the image, appearing as if the computer can “see” the world around it. And it doesn’t stop there. Images can be combined with text to provide context or suggestions for what to focus on in the images.
This advancement offers plenty of revolutionary potential for those with vision impairments and applications like autonomous vehicles, smart home devices, etc. However, with more capability, comes the added need for responsibility, and this is where some of our critiques and concerns begin.
Should AI be Open Source?
The biggest, most immediate pressing concern for those in the AI space is how “closed source” these large-scale models are becoming. With many early adopters and tight roots in academia, this is the inverse of the best practices discussed.
OpenAI has gone above and beyond just feel-good values, sharing in its announcement blog post that they are driven to “advance digital intelligence in a way that is most likely to benefit humanity as a whole, unconstrained by a need to generate a financial return.” However, recent efforts have concerned machine learning ethicists, experts, researchers, and their own internal Red Team.
Despite previous efforts and claims of transparency – from not only OpenAI but also stakeholder Microsoft – this latest foundational model release, GPT-4, is the least transparent yet. With no transparency on model size, optimization, or even dataset due to “both the competitive landscape and safety implications…” one can be forgiven for questioning the true integrity of these large-scale models and the organization.
Unfortunately, this seems to be more than just a one-off instance but rather a trend with OpenAI as of late. As reported in The Verge — the organization moved from a nonprofit to a capped-profit organization to secure money from Microsoft. In that same Verge article, Ilya Sutskever, OpenAI’s chief scientist and co-founder, stated:
“…AI — AGI — is going to be extremely, unbelievably potent, then it just does not make sense to open-source. It is a bad idea... I fully expect that in a few years, it’s going to be completely obvious to everyone that open-sourcing AI is just not wise.”
Ben Schmidt, the VP of information design at Nomic AI, said it best in a Twitter thread share on the day of GPT-4’s release:
“I think we can call it shut on ‘Open’ AI: the 98-page paper introducing GPT-4 proudly declares that they’re disclosing *nothing* about the contents of their training set,”
Closing down references and resources is only one area of concern for members.
Evaluating AI Risk
One more provoking topic in the OpenAI's GPT-4 paper was what their Red Team showed. For context, an AI Red Team is composed of people who are adversaries, attempting to exploit model vulnerabilities and providing feedback from the adversary's perspective. Red Teams are typically employed to determine the risks of AI workflows and models, including "power-seeking behavior." Think of the Black Mirror-esque Terminator tendencies portrayed in science fiction horror stories we have all come to fear over time.
The technical report shares "capability improvements relevant to some of the factors that limited the earlier models power-seeking abilities." The model was tested to understand better its liabilities and risk with power-seeking behavior, including its ability to conduct phishing attacks, replicate itself on another server, hide traces of itself, and more.
The report includes a cryptic message mentioning the Red Team that worked on the project. These teams and their workflows have been described as “learning with catastrophes” or seeing if the worst-case scenarios are possible within a given set or framework.
Specifically — reading the report, many experts (including the authors of this blog post) had strong reactions after seeing the following in the technical report;
"Participation in this red teaming process is not an endorsement of the deployment plans of OpenAI or OpenAI’s policies..." and a sequence of 42 names, presumably with release reservations.
This disclosure, in combination with the vagueness of the overall academic report, was heavily critiqued by experts, including the CEO of Lightning.ai and creator of PyTorch Lightning, William Falcon. In one article published in Venture Beat, he stated:
“… if this model goes wrong, and it will, you’ve already seen it with hallucinations and giving you false information, how is the community supposed to react? How are ethical researchers supposed to go and actually suggest solutions and say, this way doesn’t work, maybe tweak it to do this other thing?
The community’s losing out on all this, so these models can get super-dangerous very quickly, without people monitoring them. And it’s just really hard to audit. It’s kind of like a bank that doesn’t belong to FINRA, like how are you supposed to regulate it?”
Across the board, while the technology itself is exciting, we’re left with a conflicting precedent. We’re no longer embracing transparency and the scientific rigor that made these innovations possible in the first place. For many academics and early innovators, the art and integrity behind these innovations have been lost.
The integrity of the process has caused more skepticism than ever before. One could even question the amount of peer review or oversight that went into the report, with the following; "GPT-4 was used for help with wording, formatting, and styling throughout this work.”
Real Talk: Truths about ML
There’s no denying that GPT-4 and large models akin to it are on the bleeding edge of innovation. We’d be remiss if we didn’t acknowledge the work it takes to create models like this. However, the industry must acknowledge the shoulders of the giants we stand on.
Unfortunately, the giants in our peripherals are leading us to have more cause for concern than before. Simultaneously — Microsoft laid off the entire ethics and society AI team, this team — according to reporting by The Verge, “has been working to identify risks posed by Microsoft’s adoption of OpenAI’s technology…”
A turning point in computational history?
For many, this is a significant shift from how the AI and ML industry has operated previously. Historically and contextually, AI and ML research has primarily benefited from working and performing in an open and transparent environment. Academics, legacy computational scientists, and even early researchers in human-computer interaction have all benefited through collaborative efforts, typically through open source computing.
Ever since the rise of the personal computer in the late 1970s, we’ve increasingly thought about our roles as humans as we interact with the world around us. Human-computer interaction (HCI) started as a relatively isolated field and only took off in leaps and bounds when we embraced the tools as a collaboration medium. As our relationship with computers intensifies, it is crucial that we disseminate what is made public and what is made participatory, along with the reasons why.
This distinction between public and participatory will dictate the types of tools we create next. How the latest evolution of computational tools are crafted moves the ethical solution toward the participatory. It is imperative that we lean on the participatory side to reduce inequality, increase accessibility, and for public accountability and safety.
Conclusion: It comes down to data.
Referring back to the interview with William Falcon posted in Venture Beat, it’s not the innovation or the models we’re fearful of. Some ways to be competitive don’t include keeping so much shrouded in secrecy. As Falcon states:
“At the end of the day, it’s going to come down to what data you have, not the particular model or the techniques you use. So the thing they can do is protect the data, which they already do. They don’t really tell you what they train on. So that’s kind of the main thing that people can do. I just think companies in general need to stop worrying so much about the models themselves being closed-source and worry more about the data and the quality being the thing that you defend.”
Reflecting on the role that data labeling and Label Studio play in the midst of these innovations, we’re pretty excited about what the future holds and looking forward to further innovation in these areas as well.
Additionally, we see data integrity and the role of dataset development in any machine learning model process as absolutely essential to the production and development of new large-scale models. No matter your workflow or end goals, having a solid base to start from with a high-quality dataset and pre-processing routine can drastically impact model development.
For the sake of openness and to ensure the reduction of harm and possibilities for damage to be caused, we must prioritize dataset development, a strong belief in the good that the public commons can bring, and enable open access and participation in the development of further innovation.