Scaling AI Starts with Smarter Data Labeling Jobs
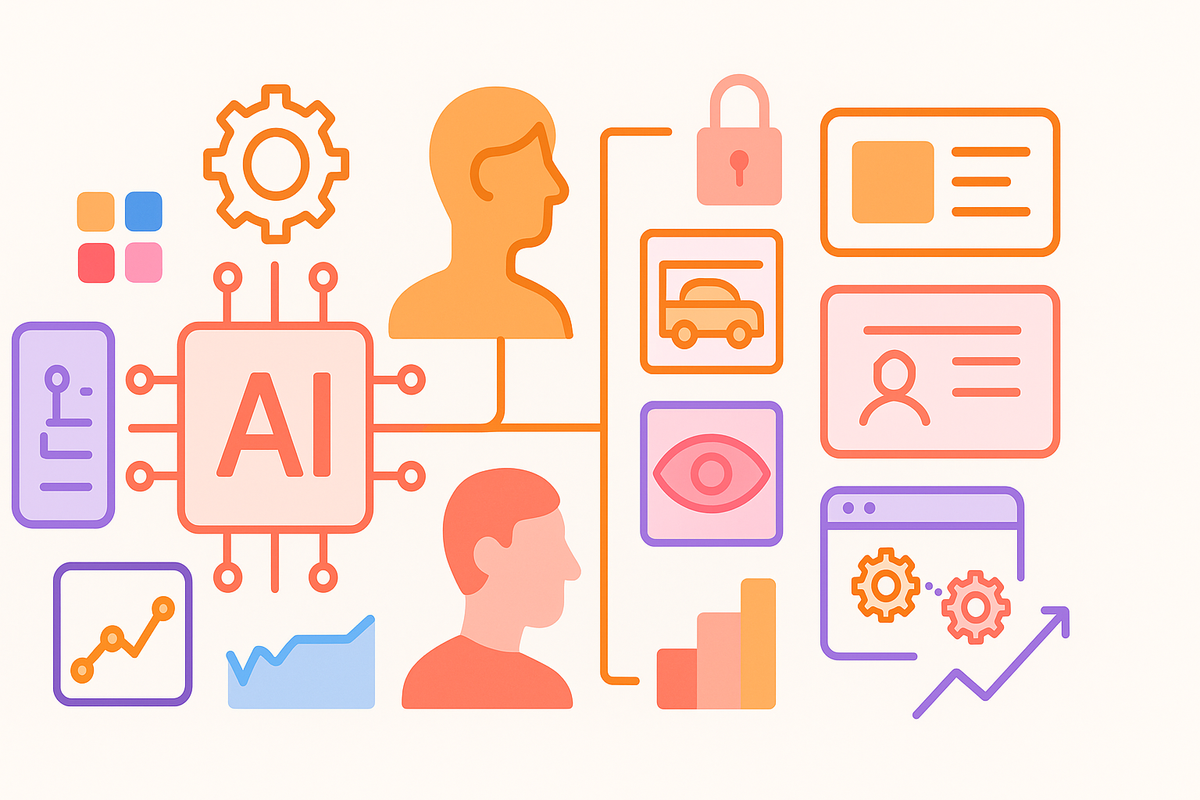
Scaling AI projects isn’t just about better models anymore, it’s about how strategically you manage and execute data labeling jobs. Whether you’re building a computer vision pipeline or training a large language model, the difference between success and failure often comes down to how you label your data and who you trust to do it.
Our work developing Label Studio has taught us that great data labeling doesn’t happen by accident. It happens when you bring together the right people, processes, and tools.
Why Specialized Data Labeling Roles Are Non-Negotiable
Modern AI is hungry for high-quality, diverse, and accurately labeled data. Without it, even the most advanced algorithms stumble. But when labeling is treated as a core function—not an afterthought, teams move faster, deliver better models, and surface clear metrics on data quality and project health.
Done right, structured labeling teams drive:
- Reliable model performance, thanks to consistent, rich training data
- Operational speed and scalability, through smart workflows and automation
- Visibility into data quality, with actionable review pipelines and feedback loops
- Compliance and security, by ensuring sensitive data is handled correctly
Success here doesn’t just come from hiring more annotators. It comes from building a team around five critical specialties.
Five Essential Jobs That Make (or Break) Data Labeling
At the heart of every AI breakthrough are the people who made the training data possible. Here’s how smart teams organize the work:
1. Precision Annotators Not all labeling is simple. Complex tasks like pixel-level segmentation, temporal video alignment, or fine-grained text classification demand specialized attention. These annotators do far more than draw boxes, they adapt to different data types, handle edge cases, and maintain consistency even under changing guidelines.
2. Domain Experts When context matters, domain specialists step in. Whether it's clinicians labeling radiology images or robotics engineers annotating LIDAR point clouds, these experts translate niche knowledge into precise labels that generalists can’t match. Label Studio supports these workflows with rich templates and validation rules that ensure domain knowledge is captured properly.
3. Quality Assurance Engineers Accuracy isn’t guaranteed, it’s enforced. QA roles design multi-stage review pipelines, monitor metrics like inter-annotator agreement, and surface systemic labeling errors before they compromise model performance. Tools like Label Studio’s agreement dashboards help teams track review outcomes in real time and catch issues early.
4. Workflow Engineers and Tooling Specialists Behind every efficient annotation pipeline is someone tuning the machine. From customizing interfaces to integrating ML-assisted pre-labeling, these engineers make labeling scalable—and less painful.
5. Workforce Managers As datasets grow, managing people becomes as important as managing data. Workforce leads handle onboarding, permissions, productivity monitoring, and compliance. It’s what allows distributed teams to scale without sacrificing quality or security.
Together, these five roles transform labeling from a cost center into a strategic advantage.
What High-Performing Data Labeling Teams Do Differently
Having the right people is only half the equation. The best teams we work with also:
- Write clear, evolving guidelines that annotators and reviewers trust
- Automate the obvious, but keep humans in the loop where nuance matters
- Tighten feedback loops between annotators, QA, and engineers
- Measure everything—coverage, agreement, error rates—to drive continuous improvement
And most importantly, they treat labeling as a living system, not a one-time setup.
HumanSignal’s Perspective: Technology Should Empower Humans
At HumanSignal, we believe that tools should lift teams up, not replace them. That’s why Label Studio is built around flexibility, extensibility, and transparency. It’s why we support:
- Multi-modal labeling for any AI use case
- Deep customization for your workflows, not ours
- Quality assurance as a first-class feature, not an afterthought
- Seamless integrations into your ML stack
We built Label Studio to help data science teams own their labeling workflows from end to end.
Future-Proofing Your Data Labeling Strategy
The future of AI belongs to teams that label smarter, not just faster. Building that future means:
- Prioritizing domain expertise alongside annotation scale
- Embracing cross-team collaboration for quality control
- Automating repetitive work while keeping judgment calls in human hands
- Investing in flexible, future-ready platforms that evolve with your needs
Data labeling is no longer just a task. It's a discipline. And teams that treat it that way will power the next generation of AI innovation.
Ready to transform how your team labels data? Get started with Label Studio today or reach out, let’s talk about how to future-proof your data strategy.
Frequently Asked Questions
Data Labeling Jobs: Frequently Asked Questions
1. What are data labeling jobs in machine learning?
Data labeling jobs involve tagging raw data—like text, images, audio, or video—with meaningful annotations that machine learning models use to learn. These jobs range from drawing bounding boxes around objects to classifying sentiment in a sentence, and they’re critical for training high-quality AI systems.
What roles are involved in a data labeling team?
A high-functioning data labeling team typically includes precision annotators, domain experts, quality assurance engineers, workflow engineers, and workforce managers. Each plays a distinct role in ensuring labeling accuracy, efficiency, and scalability.
Why are specialized data labeling roles important?
Specialized roles help ensure that labeled data is accurate, consistent, and usable for real-world AI applications. For example, domain experts provide context that general annotators might miss, while QA engineers enforce accuracy through review pipelines and error monitoring.
4. How can teams improve the quality of data labeling jobs?
Teams can improve quality by writing clear guidelines, building feedback loops between annotators and reviewers, using pre-labeling models for efficiency, and continuously measuring agreement, coverage, and error rates.
5. What tools support high-quality data labeling jobs?
Label Studio supports high-quality labeling by offering customizable interfaces, multi-modal support, quality assurance features, and integrations with existing ML stacks. Label Studio can help teams manage complex workflows while maintaining data integrity.